The methods used to conduct this living literature review of Covid-19 misinformation interventions were informed by the Preferred Reporting Items for Systematic Reviews and Meta-Analyses (PRISMA), Cochrane’s guide on Living Systematic Reviews (LSR), and the scoping review framework proposed by Arksey and O’Malley. The latter details a process wherein you: 1) specify the research questions 2) identify the relevant literature 3) select the studies 4) extract, map and chart the data and 5) summarize the findings and report the results.
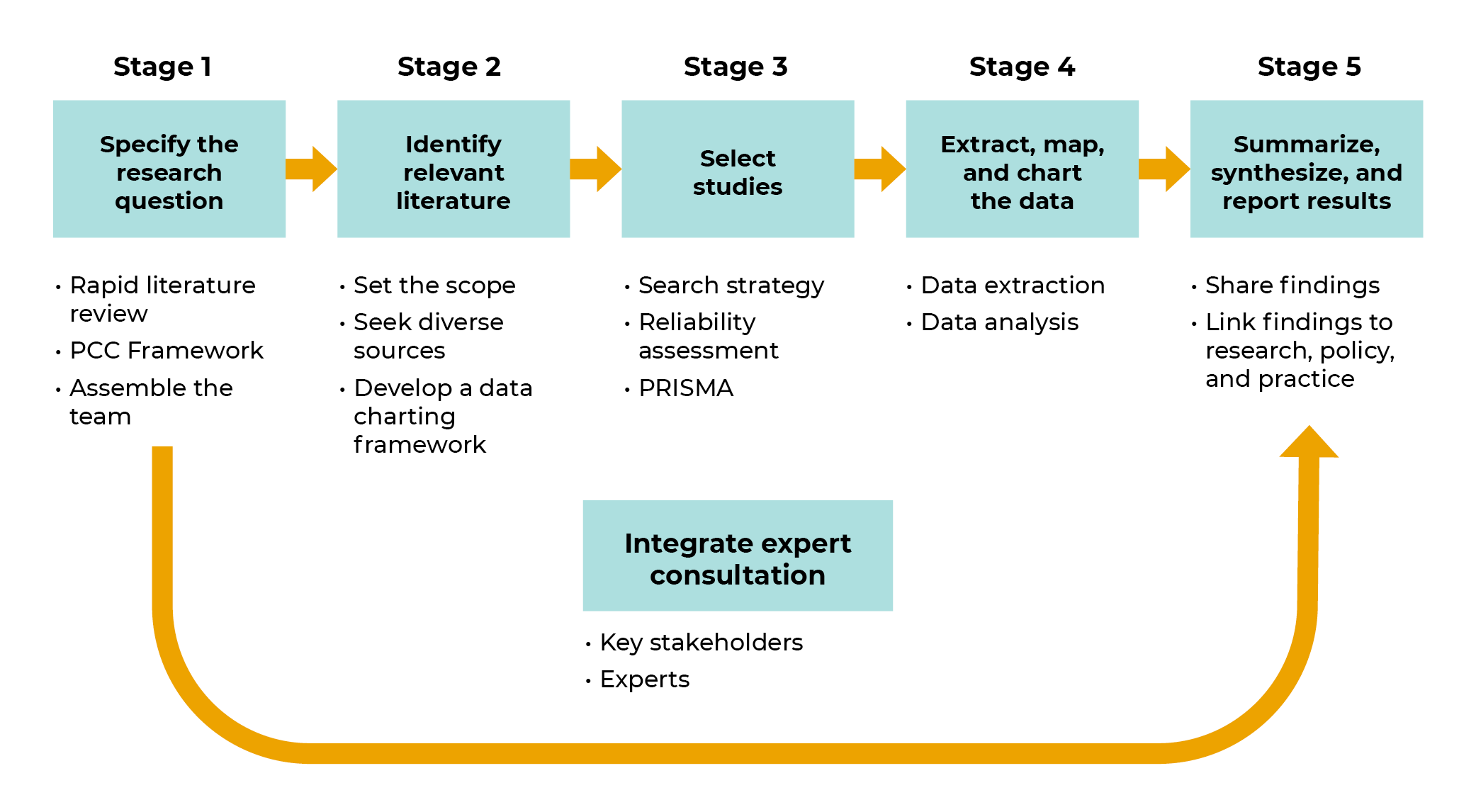
Overarching questions included:
- What kinds of interventions were tested against Covid-19 misinformation?
- To what extent did Covid-19 interventions help in protect individuals against the influence of misinformation?
- How do Covid-19 misinformation studies measure effectiveness?
Overarching questions included:
- What kinds of Covid-19 misinformation were the interventions tested against?
- To what extent were different interventions tested against different kinds of Covid-19 misinformation?
- Where and when did studies take place?
Overarching questions included:
- What major gaps still exist in the research about the effectiveness of Covid-19 misinformation interventions?
- What should future research prioritize?
- How can the results from this review inform research about health misinformation interventions more broadly?
To search for literature relevant to Covid-19 misinformation interventions, we used a combination of strategies. We first created a detailed list of boolean queries related to misinformation interventions. The queries were both deductive — they borrowed language from recognized misinformation interventions — and inductive — they were made up of open-ended terms allowing for the identification of novel misinformation interventions.
It should be noted that for the sake of brevity, we use the term “misinformation” to represent many aspects of information disorder, such as myths, misconceptions, inaccuracies, infodemic, misinfodemic, falsehoods, disinformation, rumors, conspiracy theories, misleading information, or information taken out of context. These terms are reflected in our boolean queries.
We used the detailed list of Boolean queries to search the titles and abstracts of papers in multiple on- line databases. Our search strategy resulted in papers where Booleans matched either the title or the abstract. Databases included Medline, PubMed, CINAHL, EBSCO, PsycINFO, Web of Science, Sociology Abstracts, SocINDEX, Embase, EuropePMC, Open Science Framework, JMIR Preprints, and the ACM Digital Library. We included intervention-based (experimental and quasi-experimental) peer-reviewed studies, grey literature (such as Organization for Economic Cooperation and Development papers), conference papers, and preprints assessing the effectiveness of interventions against the effects of COVID-19 misinformation on human participants that were published online between January 1, 2020, and February 24, 2023. We included grey literature, conference papers, and preprints because the novelty of COVID-19 requires that decisions be informed by the best available evidence at the time.
Several reviews carried out during the pandemic that examined preprints showed that the quality of these studies did not markedly differ from that of peer-reviewed articles.
To ensure we were comprehensively searching all relevant material, we also input the booleans into the Google Scholar engine of SerpAPI. SerpAPI is a service that allows for the scraping of Google and other search engines. The combination of searching academic databases and Google Scholar turned up 4,047 papers. We amalgamated the results in the papers into a CSV format and used Python and Pandas to remove duplicates. Given how different versions of the same papers may be indexed in Google Scholar and how their titles may slightly differ slightly, a pure string matching and removal approach was deemed insufficient to remove all the duplicates. We thus added another layer of duplication removal using the Semantic Textual Similarity feature of SBERT. SBERT is a Python framework for sentence embeddings. We set the threshold for cosine similarity to 95% and excluded all papers (minus one version) that scored below that threshold.
A total of 121 papers met our screening criteria. Two team members reviewed the full texts of each paper, ensuring that the studies were either experimental or quasi-experimental. This resulted in fifty papers that met our inclusion criteria. Papers often included multiple studies, and within these studies, multiple interventions could be tested. Within the 50 papers, we analyzed 119 interventions as part of this review.
The screening process was conducted by two members of the research team and was made up of three main stages.
1) In the first stage, we vetted the titles of papers for relevance, ensuring that they related to Covid-19 misinformation interventions. Where titles were unclear, we also investigated paper abstracts for further cues signaling relevance. We filtered out news articles — Google Scholar results can be noisy, lumping academic studies with news articles.
2) In the second stage, we revisited the remaining papers ensuring they met our eligibility criteria.. We removed descriptive, theoretical and opinion-based papers as well as systematic reviews, scoping studies and meta-analyses.
We only included on papers that tested an intervention against Covid-19 misinformation stimuli. Some studies included misinformation that was non-Covid-19 related. In such cases, we focused only on the effects of the intervention on the Covid-19 misinformation stimuli.
3) With the remaining papers, we conducted both backwards and forwards citation chaining, and repeated stages 1-2 to ensure study relevance.
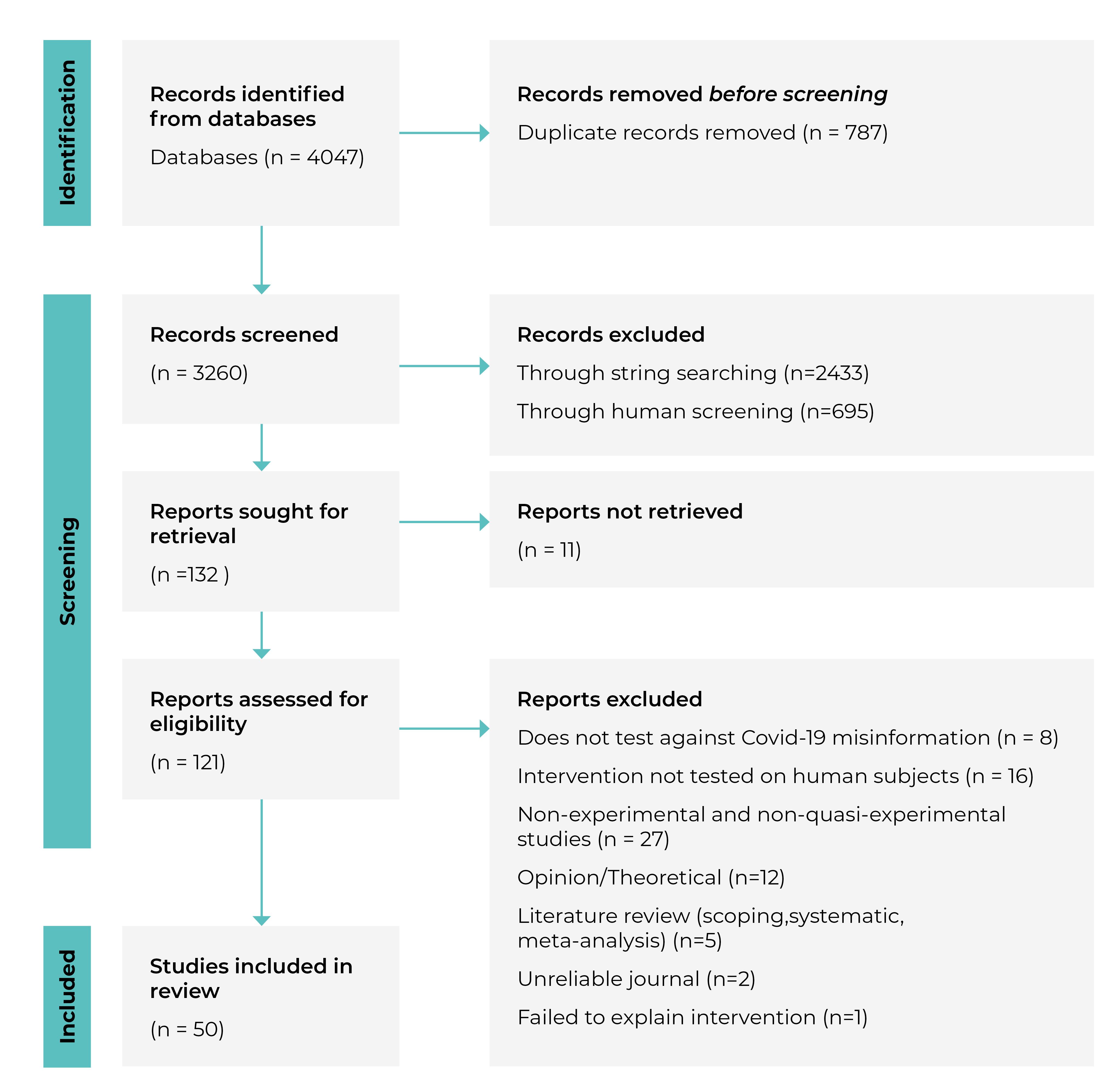
PRISMA 2020 diagram
The research team first determined a list of data fields to extract from the relevant studies. Criteria for inclusion were twofold: 1) data that would help the research team address the overall aims and research questions of the review and 2) data that could be useful for public health experts, researchers, journalists and community organizations to conduct research on Covid-19 misinformation interventions, inspire further research about misinformation interventions and inform policy decisions. We originally extracted 123 data fields. Those fields (excluding the outcome measures) are as follows:
academic_field, research_methods, medium_misinfo, date, proactive_reactive, intervention_modality_image_static, university_affiliation, study_number, intervention_modality_audio, study_timeline, sample_characteristics, journal_ranking, intervention, modality_image_static, misinfo_topic, countries_tested, language, information_medium_intervention, modality_text, country_of_authorship, sample_size, time_elapsed, debriefing_methods_detailed, recruitment_platform, study_design, intervention_number, study_longitudinal, platform_emulation, length_delay, authors, modality_video, debriefing_mentioned, intervention_modality_video, tags, journal_conference, article, doi_link, misinformation_tested, intervention_modality_text, delay_misinfo_intervention, source_type, citations, misinformation_used, intervention_modality_symbol, key_findings, abstract, relevant_intervention_details, channel_of_intervention, channel_of_misinfo, recruitment_timeline, title, modality_audio, and long_countries_tested.
The extraction of relevant data was carried out by two members of the research team and in two stages. They first individually parsed all the selected papers, extracting all the relevant data from each paper and storing it in their own personal spreadsheet whose columns were labeled with the appropriate fields. After completing this first phase, the two team members then went row by row and field by field comparing their respective results for agreement and inputting those data where there was agreement into a master spreadsheet. Where discrepancies existed, team members revisited the relevant paper, discussed the disagreement and agreed on which data would be added to the master database. If there was still disagreement after this deliberation, a third researcher was consulted. The final data were stored in four separate but relatable data tables.
To map the different Covid-19 misinformation interventions, we used, to the extent possible, pre-existing definitions of interventions found in the misinformation literature which best fit the interventions discussed in the 50 studies. In some cases, we had to accommodate interventions that weren’t explicitly detailed in the extant literature. “Monetary incentives” and “priming” are particular examples of interventions we identified in this review that had previously received little attention in the misinformation literature. As such, the process was both deductive — we borrowed terms and definitions from existing intervention taxonomies — and inductive — we identified and classified interventions that had previously received little to no attention. Current taxonomies of misinformation interventions are not perfect; definitions could be tightened and refined. In some instances, interventions may not fall perfectly into one intervention type or another. Such definitional ambiguities make strict classifications difficult and we acknowledge the limitations of adopting a rigid, bucketed approach. However, we hope that bringing these definitional imperfections to light may inspire more conversation about and action around concretizing terminology about misinformation interventions.
In order to evaluate the extent to which different Covid-19 misinformation interventions were effective, we first had to map all of the outcome variables identified in the 50 studies. Outcome measures were included if they were clearly defined in the Methods sections of each respective study and if they were established as direct outcomes of the intervention. Examples of outcome measures include: belief in misinformation, intent to share misinformation, and intent to practice Covid-19 preventative behaviors among others. Some studies included mediation models to better understand the relationships of different variables. Where a study showed an outcome measure to be an indirect effect of the intervention through mediation models, the details of these measures were detailed under the column relevant_intervention_details; these measures were not coded independently. We initially identified 65 different outcome measures across the 50 articles; these included long-term measures, which we coded separately. For example, belief in misinformation and long-term belief in misinformation were coded as two separate outcome measures, despite them sharing the same scales. Through a process of iterative grouping, where we closely inspected the scales used to assess each outcome measure (e.g. perceived credibility was measured using a Likert scale from 1 (not at all credible) to 5 (very credible)), and inductive organizing (e.g. outcome measures closely resembling each other such as perceived accuracy, perceived credibility and perceived veridicality were merged into the same outcome measure) we ended up with 47 separate outcome measures.
Borrowing from Guay et al. (2022), we coded the direct outcomes of the interventions as either helpful, no_effect or harmful. Whether or not an outcome was coded as helpful or harmful depended on how the intervention impacted that particular outcome across the entire sample population and only if the effect was statistically significant at p <0.05. We did not segment the outcome measures by different demographic slices or confounding variables.. For example, if a debunk had a positive impact on reducing belief in Covid-19 misinformation across the entire population and its effect was significant we coded that as helpful. If subsequent analyses revealed that among conservatives with low levels of conscientiousness the debunk had no effect, the outcome variable belief_in_misinformation would still be coded as helpful. However, within the column relevant_effectiveness_details those mediating factors (conservatives with low levels of conscientiousness) would be detailed. Interventions that had non-significant outcomes were coded as no_effect. A meta-analysis of all the interventions was deemed infeasible due to the wide variety of interventions studied, the different misinformation stimuli tested in studies, and the extensive use of different outcome measures.
“Covid-19 misinformation” can mean many different things. It may refer to vaccine misinformation, false treatments, misleading statistics, or 5g conspiracy theories, among many other examples. To assess the extent to which the interventions were tested against different topics of misinformation, we first extracted all the Covid-19 misinformation claims (referred to as misinformation stimuli) shown to participants in the studies. Rarely did articles present the misinformation stimuli in the body of the article. Most often the misinformation examples were tucked away in supplementary material sections that were attached to the article, or hosted on OSF. Thus the identification of misinformation stimuli presented to participants in these studies required the research team to sift through droves of supplementary material and appendices to identify stimuli. Given this process, it’s possible that we missed certain examples of misinformation in the data collection process. Nonetheless, to the best of our knowledge, this is the first study to systematically extract and explore the misinformation stimuli from Covid-19 misinformation intervention studies.
After extracting the Covid-19 misinformation examples, we then had to decide how to categorize the stimuli. Categorizing the misinformation stimuli under specific topics would more clearly show the extent to which the interventions had been tested on different examples of Covid-19 misinformation and where possible gaps exist. To identify relevant topics we revisited 12 studies conducted at different points of the pandemic that aimed to classify different topics of misinformation. These studies were segmented into two groups: 1) studies that classified general topics of Covid-19 misinformation and 2) studies that classified Covid-19 vaccine misinformation.
Covid-19 Misinformation
Brennen JS, Simon FM, Howard PN, Nielsen RK. Types, sources, and claims of COVID-19 misinformation [Internet]: University of Oxford; 2020 [Available from: https://reutersinstitute.politics.ox.ac.uk/types-sources-and-claims-covid-19-misinformation.
Covid-19 Misinformation
Charquero-Ballester M, Walter JG, Nissen IA, Bechmann A. Different types of COVID-19 misinformation have different emotional valence on Twitter. Big data & society. 2021;8(2):205395172110412.
Covid-19 Misinformation
Hansson S, Orru K, Torpan S, Bäck A, Kazemekaityte A, Meyer SF, et al. COVID-19 information disorder: six types of harmful information during the pandemic in Europe. Journal of risk research. 2021;24(3-4):380-93.
Covid-19 Misinformation
Mohammadi E, Tahamtan I, Mansourian Y, Overton H. Identifying Frames of the COVID-19 Infodemic: Thematic Analysis of Misinformation Stories Across Media. JMIR Infodemiology. 2022;2(1):e33827-e.
Covid-19 Misinformation
Nissen IA, Walter JG, Charquero-Ballester M, Bechmann A. Digital Infrastructures of COVID-19 Misinformation: A New Conceptual and Analytical Perspective on Fact-Checking. Digital journalism. 2022;10(5):738-60.
Covid-19 Misinformation
Islam MS, Sarkar T, Khan SH, Mostofa Kamal A-H, Hasan SMM, Kabir A, et al. COVID-19-Related Infodemic and Its Impact on Public Health: A Global Social Media Analysis. The American journal of tropical medicine and hygiene. 2020;103(4):1621-9.
Covid-19 Misinformation
Charquero-Ballester M, Walter JG, Nissen IA, Bechmann A. Different types of COVID-19 misinformation have different emotional valence on Twitter. Big Data & Society. 2021 Jul;8(2):20539517211041279.
Covid-19 Vaccine Misinformation
Smith R, Cubbon S, Wardle C. Under the surface: Covid-19 vaccine narratives, misinformation and data deficits on social media 2020 [Available from: https://firstdraftnews.org/wp-content/uploads/2020/11/FirstDraft_Underthesurface_Fullreport_Final.pdf?x21167.
Covid-19 Vaccine Misinformation
Muric G, Wu Y, Ferrara E. COVID-19 Vaccine Hesitancy on Social Media: Building a Public Twitter Data Set of Antivaccine Content, Vaccine Misinformation, and Conspiracies. JMIR public health and surveillance. 2021;7(11):e30642-e.
Covid-19 Vaccine Misinformation
Lurie P, Adams J, Lynas M, Stockert K, Carlyle RC, Pisani A, et al. COVID-19 vaccine misinformation in English-language news media: retrospective cohort study. BMJ open. 2022;12(6):e058956.
Covid-19 Vaccine Misinformation
Jamison A, Broniatowski DA, Smith MC, Parikh KS, Malik A, Dredze M, et al. Adapting and Extending a Typology to Identify Vaccine Misinformation on Twitter. American journal of public health (1971). 2020;110(S3):S331-S9.
Covid-19 Vaccine Misinformation
Skafle I, Nordahl-Hansen A, Quintana DS, Wynn R, Gabarron E. Misinformation About COVID-19 Vaccines on Social Media: Rapid Review. Journal of medical Internet research. 2022;24(8):e37367-e.
Topics overlapped across the studies. Through an iterative process, different topics from these studies were selected based on their relevance to the Covid-19 misinformation found within the 50 papers. The process of deductively categorizing content under specific topics comes with limitations. Even the best-trained human coders and machine learning classifiers get it wrong. There are always certain pieces of content that don’t fit squarely into one category or another; they may contain elements of several categories. Recognizing these limitations and in an attempt to minimize human error, topics were chosen not only for their relevance to the data we extracted from the studies but also for their mutual exclusivity. In order to ensure the accuracy and reliability of the results of the two coders, we used the Cohen’s Kappa inter-rater reliability test, which ranges from 0-1 with higher numbers equating to higher levels of reliability. Both coders independently coded 90 instances of Covid-19 misinformation. The Kappa test resulted in a score of 0.9, suggesting a high level of reliability. Both researchers then coded the remaining pieces of Covid-19 misinformation from the studies. Results were compared between the two coders and where discrepancies existed, coders discussed the piece of content until a final topic was decided on by both researchers. In instances where there was still disagreement, a third researcher was consulted.